Question: What are the two types of statistical inference we have studied so far?
Two Types of Statistical Inference
- Confidence Intervals
- Tests of Significance (Hypothesis Tests)
Question: What are the assumptions of the procedures we have used so far?
Another Question: Are these always reasonable assumptions?
Do we Have an SRS?
Here is a bit of statistical wisdom for you: no amount of fancy analysis can correct bad data.
We know several sure-fire ways of collecting bad data.
We also know that sometimes there are more suble ways of collecting a flawed data set.
Can you recall any?
Fact: When you use statistical inference, you are acting as if your data are a random sample or come from a randomized comparative experiment.
Consequence: If your data don’t come from a random sample or a randomized comparative experiment, your conclusions may be challenged.
We may restate the above consequence as a savvy citizen fact....
Savvy Citzen Fact: If someone else's data doesn’t come from a random sample or a randomized comparative experiment, you may challenge their conclusions.
Some Cautions when Wielding the Mighty Power of Statistical Inference
Confidence Intervals
The margin of error of a confidence interval accounts only for random sampling error.
The margin of error DOES NOT account for flawed sampling design, or practical difficulties such as nonresponse and undercoverage.
Some Cautions when Wielding the Mighty Power of Statistical Inference
Hypothesis Testing
- Don't place too much emphasis on levels of significance. $\alpha=0.05$ is a common standard, but don't take too much stock in it. When evaluating a study, always insist on a $p$-value.
- Statistical significance does not tell us whether an effect is large enough to be important. That is, statistical significance is not the same thing as practical significance.
- Beware Multiple Analyses. Running one test and reaching the 5% level of significance is reasonably good evidence that you have found something. Running 20 tests and reaching that level only once is not.
Some Cautions when Wielding the Mighty Power of Statistical Inference
...and one more thing.
Just because you fail to reject the null hypothesis $H_0$ doesn't mean that $H_0$ is necessarily true. It only means your sample doesn't provide enough evidence to reject $H_0$.
Even a large effect can fail to be significant when a test is based on a small sample.
Planning a Study
Big Question: how big does my sample have to be in order to get a desired margin of error?
Planning a Study
Recall: The general for of our $z$ confidence interval is $$\bar{x} \pm z^{*}\frac{\sigma}{\sqrt{n}}$$ Do you remember what the margin of error is?
Planning a Study $$n=\left(\frac{z^* \sigma}{m}\right)^2$$ Example: Suppose the body mass index (BMI) of all American young women follows a Normal distribu- tion with standard deviation $\sigma = 7.5$. How large a sample would be needed to estimate the mean BMI $\mu$ in this population to within $\pm 1$ with 95% confidence?
Example: You are a detective that investigates gaming fraud. Suppose you want to calculate a confidence interval for the mean of the die that Sleazy P. Martini was rolling. How many rolls would you need to record in order to estimate the mean $\mu$ of within $\pm 0.5$ with 99% confidence?
Planning a Study: Tests of Significance
Power: The power of a test of significance is it's ability to detect a difference in a population parameter IF SUCH A DIFFERENCE EXISTS.
We may state this as a probability:
The power of a test of significance is the probability that the test will reject $H_0$ given that $H_a$ is true. $$\mbox{Power}=P(\mbox{Reject $H_0$}|\mbox{$H_a$ is true})$$
Possible Errors in Tests of Significance
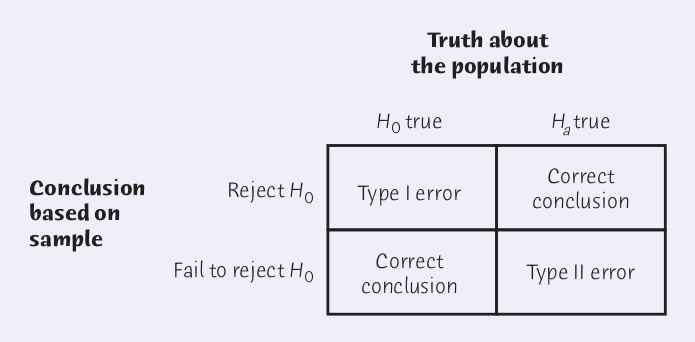
$$P(\mbox{Type I Error})=\alpha$$ $$P(\mbox{Type II Error})=1-\mbox{Power}$$
Pop Quiz: Will power increase or decrease with sample size?
Increasing the size of the sample increases the power of a significance test.
Burning Question: How do we calculate the power of a test?
Drum roll please.........
Answer: Use software.